Why is data governance so important for finance teams?
Data governance in finance is about two things: identifying the most relevant data and setting rules for its use. Without these guardrails, even clean data can be unreliable.
Strategic financial planning and decision-making relies on strong foundations—and well-governed, structured, and high-quality data is part of that foundation. Data governance ensures that information is pulled from the right sources, categorized correctly, and put into context so finance teams can make accurate forecasts, avoid compliance risks, and minimize financial losses.
Take annual recurring revenue (ARR), for example. If contracts aren’t categorized correctly as recurring or non-recurring, ARR reporting will be inaccurate. That skews forecasts, misguides decision-making, and impacts investor confidence. But when finance teams have a structured, well-managed data foundation, they can confidently maintain security, improve forecasting, and make strategic decisions that offer an edge over their competitors.
Data governance vs data validation
While data governance and data validation are related, they serve different purposes within finance teams.
- Data governance is the overarching framework that defines how financial data is collected, stored, accessed, and used. It establishes policies, roles, and standards to ensure data integrity, security, and compliance across an organization.
- Data validation is a key process within data governance that ensures data accuracy, completeness, and consistency. It involves checking data against predefined rules, identifying discrepancies, and correcting errors before the data is used for reporting or analysis. Without proper validation, even well-governed data can contain errors that impact financial planning and forecasting.
For example, a finance team using AI-driven finance tools can enforce governance policies that dictate which datasets should be used for financial reporting. Meanwhile, automated validation processes ensure that every transaction recorded in those datasets is accurate, preventing misclassifications that could lead to reporting errors. Together, governance and validation create a structured, error-free environment for financial analysis.
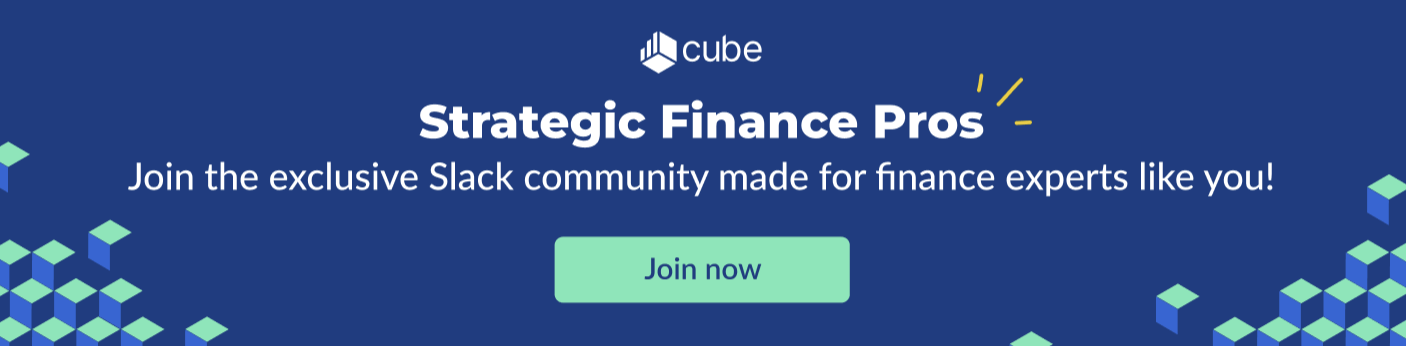
What is AI in data governance?
AI in data governance can refer to two things. One: how artificial intelligence can transform manual data management into automated, intelligent processes. AI-powered data governance applies machine learning algorithms to validate, organize, and protect financial data in real time—creating a more reliable foundation for decision-making.
And two: For AI to work effectively, it needs well-governed data. Poor governance can lead to biased models, inaccurate predictions, and compliance risks that undermine the AI’s potential.
Machine learning models identify data patterns and relationships at scale, while Natural Language Processing (NLP) converts unstructured financial information into searchable, analyzable formats. This allows finance teams to access precise data faster and make more informed decisions. However, if AI is fed inconsistent, incomplete, or misclassified data, its outputs can be misleading, resulting in flawed financial strategies.
So while AI can enhance governance by automating processes like data validation and anomaly detection, it also depends on strong governance policies to ensure accuracy, consistency, and compliance.
Benefits of using AI in data governance
AI automates data governance processes, reduces errors, and improves compliance by cleaning and structuring data. Here’s a closer look at the tangible benefits of AI data governance that finance teams enjoy.
Stronger compliance and security
AI-powered access controls monitor data interactions and alert teams to unauthorized activity. The system detects patterns that may indicate fraud or compliance issues early so you can manage risks before they become bigger issues. Advanced encryption and masking protocols ensure sensitive financial data stays protected, with access limited to authorized personnel.
Reduced manual effort and human error
AI reduces the burden of repetitive data management tasks, allowing finance professionals to focus on analysis rather than data cleanup. Automated data validation ensures that financial records are accurate and consistent, while machine learning algorithms help detect discrepancies before they lead to reporting errors.
For example, if you’re using AI tools for financial planning and analysis (FP&A), the tech can handle the systematic tasks: categorizing transactions, resolving discrepancies, and maintaining governance policies across datasets. The automation reduces errors while allowing analysts to concentrate on strategic planning.
Real-time insights for better decision-making
AI provides immediate access to accurate and up-to-date financial data. Financial consolidation features automatically update reports as new information flows into connected financial, human resources (HR), and enterprise resource planning (ERP) systems. Live dashboards reflect actual performance metrics, which empowers finance leaders to respond quickly to market shifts.
With AI-driven governance, finance teams no longer need to spend hours manually reconciling reports. Instead, they can trust that their data is accurate, structured, and ready for analysis—so they can make confident, data-driven decisions in real time.
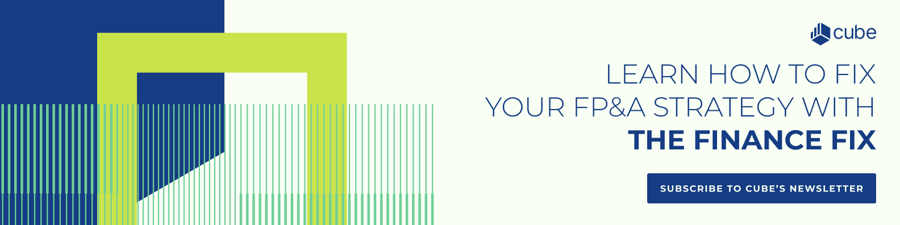
Different ways to use AI to streamline data governance processes
When implemented correctly, you can use AI in finance to create a continuous validation system that protects data integrity while reducing the time finance teams spend on oversight.
Automated data validation
Manual validation requires extensive time and resources—but AI changes that equation. AI can apply validation rules in real time across multiple data sources. When revenue numbers don't reconcile between systems, AI flags the mismatch immediately, before it impacts decisions. When expense classifications violate accounting standards, AI prevents the entry. The result? Clean, compliant data flows into reports and forecasts without constant manual review.
Error and anomaly identification
Financial data errors create ripple effects through forecasts, stakeholder communications, and compliance. AI prevents these cascading problems by monitoring data patterns and identifying deviations. For example, if transaction volumes spike unexpectedly, AI alerts teams to potential fraud risks. Or when duplicate invoices enter the system, AI stops processing before payment occurs. AI can run in the background so you can make quick fixes immediately while reducing manual oversight.
Proactive governance and scheduled audits
The shift from reactive to proactive governance means finance teams spend less time fixing data issues and more time leveraging insights for strategic decision-making. Scheduled audits ensure AI-driven governance policies remain effective, even as regulations and business needs evolve.
On a smaller scale, AI governance tools conduct continuous automated compliance checks, flagging gaps and allowing teams to update controls before they become a problem for long-term data integrity and security.
Data lineage tracking
Data lineage documents how financial information moves and transforms across systems. AI automates this documentation by creating detailed audit trails of every data point's journey. Following the ALCOA principles, which make data attributable, legible, contemporaneous, original, and accurate, data lineage tracking not only shows exactly where data originates, but also how values change over time, and who accesses information
For instance, AI tracks revenue figures from initial customer relationship management (CRM) entry through ERP processing and into financial statements. When questions arise, finance teams can trace any number back to its source. During audits and regulatory reviews, this clear lineage proves data integrity and compliance.
Dynamic data masking
Protecting sensitive financial data requires careful access control. Not everyone in the company should have unrestricted access to sensitive financial data. Governance policies should define who can view, modify, and share financial information. Clear ownership, accountability, and responsibilities allow teams to move faster without breaching compliance protocols.
Dynamic AI-powered data masking takes this framework to the next level by automatically concealing or encrypting confidential information based on user permissions and their role. In practice, a finance executive would see complete data, while analysts receive masked versions. However, as the analyst progresses in their career to become a manager, AI triggers additional security measures.
At the same time, continuous monitoring detects unusual patterns that could signal fraud or compliance risks. AI encryption responds to emerging threats, keeping sensitive financial data protected.
Predictive analytics
Predictive analytics turns historical patterns into forward-looking insights. AI accelerates this process by processing vast datasets to surface meaningful trends.
AI analyzes past revenue data to project future cash flows. Payment histories reveal which customers need proactive collection strategies. Market indicators signal potential impacts on financial performance. Each insight helps CFOs make informed decisions based on comprehensive data analysis.
Drive success with a strong AI data governance strategy
Finance teams that implement strong AI-driven data governance don’t just gain better control over their data, they build an essential foundation for strategic finance. The combination of cleaner, more accurate data and the right tools to manage and analyze it makes AI a powerful asset for financial decision-making.
By integrating AI into data governance, finance teams can streamline compliance, reduce risk, and optimize decision-making processes. The ability to automate validation, flag anomalies, and maintain structured datasets ensures that finance leaders always work with the most reliable information. However, not all AI systems are created equal. Investing in the right technology ensures finance teams can confidently leverage AI while maintaining control over their data processes.
Cube takes the first pass at your plans and reports—so you’re free to focus on the strategy that drives the business forward.
Book a demo with Cube today.
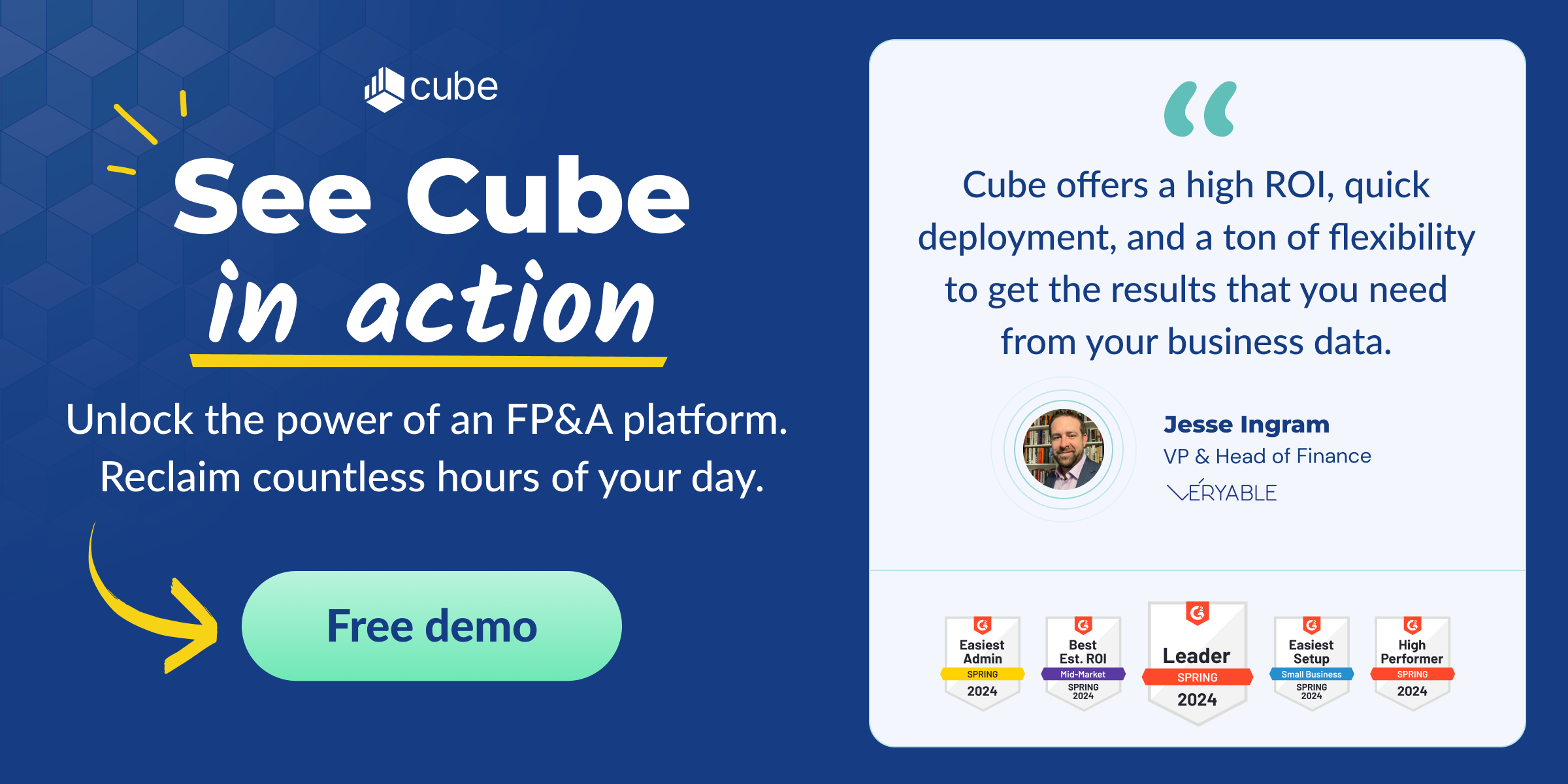