Key takeaways on AI forecasting:
- AI-driven forecasting significantly enhances the accuracy of predictions by rapidly analyzing vast and diverse data sources.
- AI introduces advanced models that outperform traditional forecasting methods in identifying patterns and trends.
- While AI offers substantial benefits, we still need FP&A professionals to create strategies, interpret data, and convert insights into real-world applications.
- Businesses that integrate AI into their forecasting processes gain a competitive edge through better resource allocation and strategic planning.
What is AI forecasting?
AI forecasting is the application of artificial intelligence and machine learning techniques to predict future financial outcomes based on historical data. Unlike traditional forecasting methods that only rely on statistical models and human judgment, AI forecasting analyzes vast datasets, identifies patterns and generates predictions to inform a finance team’s judgments.
Although the content of these forecasts could change, all of them do essentially the same job: removing as much guesswork as possible by drawing on enormous past datasets. The more data these models receive, the more accurate their forecasts.
AI forecasting is particularly powerful in handling complex scenarios where multiple variables interact, offering insights that would be difficult or extremely time-consuming to uncover using conventional methods.
Applications of AI forecasting
AI forecasting is an FP&A tool that can be applied to various industries, from manufacturing and real estate to software and healthcare. The same principles apply to all applications; algorithms analyze large amounts of data to optimize operations and stay ahead of market trends.
Let’s take a look at some major applications:
Producing reliable strategic budgets for businesses
In financial forecasting, AI gives you more accurate predictions of revenue, expenses, and cash flow for a far more confident budgeting and financial strategy. FP&A managers combine their historical financial data with external economic indicators to compare outcomes for different plans. Artificial intelligence works continuously, identifying emerging trends and anomalies that might impact future financial performance.
Optimizing supply chains to save money and time
Finance teams can use AI models to connect the dots between a vast range of historical data and map out reliable rises and falls in demand, which minimizes overstocking, understocking, and high inventory costs. They also predict the financial impact of supply chain disruptions, such as delays due to flu season, so teams can prepare to mitigate the situation. Whenever anomalies occur, FP&A teams can use algorithms to analyze the causes and add them to the model.
Predicting rises and falls in sales demands
Sales and marketing teams feed years of past consumer behavior patterns, market trends, and social media data into models to better predict fluctuations in sales. FP&A teams can therefore plan to accommodate changes in consumer demand, improve income through personalized marketing campaigns, and recommend hiring drives in time for busy periods.
They can also suggest ambitious but attainable targets for each market, rather than measuring them by the same metric.
Cross-referencing medical records for improved patient outcomes
AI forecasting allows a doctor to compare patient data with that of millions of patients all over the world and spot risk factors in seconds. When the average time spent with a physician is just over 17 minutes, this rapid analysis can point to diagnoses that could take an individual a lifetime to uncover. These efficiencies allow finance teams to redistribute budgets, focusing more on care and research than on ineffective treatments.
Streamlining consumption and management in the energy sector
AI forecasting is increasingly used by financial minds in the energy sector to predict demand and optimize how they use their resources. Factors such as weather patterns, historical energy consumption, and economic downturns can all inform energy providers on how much supply they need to provide.
This leads to more sustainable energy use, reduces waste, and supports the integration of renewable energy sources into the grid.
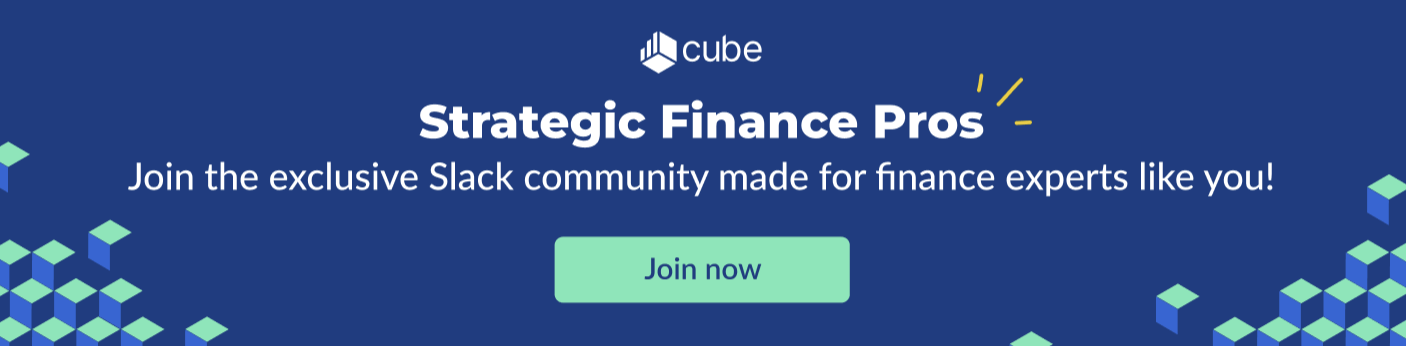
AI forecasting vs. traditional forecasting methods
Although both AI forecasting and traditional methods have the same end goal, there are qualitative and quantitative differences between the two.
For starters, traditional forecasting typically relies on statistical models that depend heavily on human intuition and historical trends. While these methods can be effective in stable environments with limited variables, they struggle to adapt to complex, rapidly changing conditions and may overlook subtle patterns in data.
AI forecasting outperforms purely human-led techniques by analyzing much bigger amounts of data in a fraction of the time. This allows artificial intelligence to look at more sources and uncover correlations that traditional methods are likely to miss. Similarly, AI models constantly update, meaning decision-makers can get an instant snapshot of real-time data, rather than relying on time-consuming calculations that might only come once a quarter.
As traditional methods often require manual data processing and model adjustments, they tend to be time-consuming and prone to error. This can be tenable—but far from desirable—for small operations, but big problems arise when you try to scale up. Because AI automates data processing, you can be more agile and grow your company without losing the accuracy and speed of your forecasting.
However, AI only serves to provide cold, hard data. Humans provide contextual understanding and industry-specific knowledge that AI models lack, so provide a strategic framework for AI to work within.
How AI forecasting works
As AI forecasting follows similar principles to traditional forecasting, many of the core elements are the same. You still need to understand what your end goals are and tweak your approaches when you spot errors. However, AI forecasting also includes a deep knowledge of which machine learning tech and what models you want to use.
1. Define forecasting goals
Your goals are the focal point of your forecasting efforts, so they need to be defined before you do anything else. It could be sales figures, demand trends, or financial performance, and you have to include a fixed timeframe to operate in.
2. Train AI models
Training AI models starts with understanding which AI technology and techniques are best for your needs.
Do you want to use machine learning algorithms to analyze historical data? Or do you want to go one step further to deep learning (DL) neural networks, which can look at far more data but are less transparent about how they come to conclusions?
In terms of models, finance teams often use regression-based models to retrospectively observe how dependent one variable is on another. However, institutions like the US Federal Reserve use a time series forecasting method known as the Autoregressive Integrated Moving Average (ARIMA) model.
Make sure to train your AI models with relevant data aligned with your business objectives to produce more accurate and actionable forecasts.
3. Process and clean data
Finance teams use data processing and cleaning to ensure input data is accurate, complete, and error-free. The process involves removing outliers, filling in missing values, and standardizing data formats to create a consistent dataset for the AI to analyze. Since inaccuracies in the data can lead to skewed predictions, cleaner data lends itself to more reliable forecasting.
4. Monitor financial models and improve AI forecasts
Even with the best planning and cleanest data, you may need to make a few tweaks to your models. Track your models' performance over time, keep an eye out for deviations or inaccuracies, and update models as soon as new data becomes available.
Your models won’t do all your work for you. Teams still need data specialists and area experts on hand to forecast successfully.
Benefits of using artificial intelligence for forecasting and planning
When implemented correctly, artificial intelligence can transform forecasting and planning with:
More accurate predictions
Algorithms can draw on far larger datasets than traditional methods at much quicker speeds. They produce informed forecasts in a vastly reduced time frame, allowing FP&A teams to make more reliable decisions and reduce costly errors. AI-driven financial planning software also refines its predictions over time so budgets stay accurate and relevant.
Improved efficiency
AI-driven forecasting automates much of the data processing and analysis involved in forecasting—two traditionally time-consuming elements. This efficiency allows you to leapfrog certain steps in your workflows and forecast efficiently.
With informed budgets in hand, you can respond to market changes and make timely decisions for a competitive edge.
Better strategic planning
Strategic planning is a critical component of any successful organization. It drives decision-making, maximizes profit, and provides the basis for long-term financial health. But… it takes a lot of time. Almost 70% of CFOs say they spend significant time on scenario planning and data analysis.
With AI, you can pass over manual tasks to algorithms, so you have more time to think strategically. Supported by AI insights, you can forecast, budget, and plan quickly and accurately.
Cost savings
AI forecasting helps to keep costs down by optimizing resource allocation, reducing waste, and minimizing the risks associated with inaccurate predictions. Automated processes and improved efficiency lower operational costs, while better forecasting accuracy helps avoid errors like overproduction or stockouts.
Over time, these cost savings can significantly improve your bottom line, making AI a valuable investment.
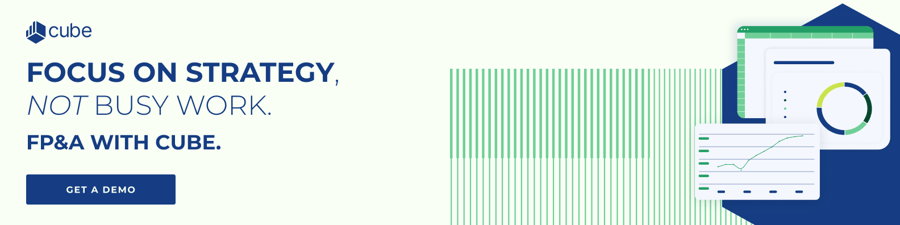
Limitations of AI-driven financial analysis
While AI forecasting offers many benefits to FP&A teams, it’s not without its challenges.
Requires vast amounts of data
The more data AI-driven financial analysis possesses, the greater its utility. But at the other end of the scale, a small amount of data can lead to inaccurate results. Companies with little historical data will struggle to learn much from the past and may instead turn to purchasing expensive financial datasets.
These can offer a rough guide, but as we’ll see in the next point, can skew predictions.
Needs accurate, complete data
Artificial intelligence still isn’t conscious—it can only work with the information it is given. While some models will ignore clear anomalies, if your data isn’t accurate or full, AI will treat it as gospel. That’s why it is essential to have a specialist who can point out inconsistencies, clean your data, and remove any duplicates to avoid decisions being made on faulty intelligence.
Creates potential forecasting biases
One of the biggest problems with forecasting as a whole is the potential for biases in data. For example, any errors in historical data in training will be brought forward by the AI models. Similarly, without human intervention, AI forecasts may repeat approaches from the past and miss out on novel breakthroughs.
Can be resource-intensive
The need for specialized hardware, software, and skilled personnel can drive up costs, particularly for smaller organizations with limited budgets. Additionally, the ongoing process of monitoring, updating, and refining AI models requires continuous time investment, which can be a strain on organizational resources over the long term.
Some of these limitations might look scary at first glance, but with purpose-built AI forecasting software, you can significantly mitigate them. AI-powered tools draw data from various sources onto one simple platform, giving you more time to think strategically. They also work within current regulations, such as SOC 2 Type 2, to keep you compliant and your data safe.
In short, there’s no need to build your models from the ground up—existing AI software offers ready-made capabilities and frameworks for you to operate in.
What to look for in AI forecasting tools
There are quite a few AI forecasting tools on the market. When making your selection, here are some of the essential elements you'll want to look for:
- Seamless integration with your existing data sources for accurate and real-time data access.
- User-friendly interfaces that make the tool accessible to non-technical users.
- Data preprocessing features to clean and validate data automatically.
- Built-in compliance to stay aligned with evolving regulatory requirements.
- Easy scalability to grow with your business and adapt to changing data needs.
- Advanced analytics and customizable forecasting models to meet goals specific to your organization.
- Strong customer support and training resources to help your team maximize the tool's potential.
Best AI forecasting tools
To give you an idea about what’s already on the market, here’s a list of the best AI forecasting tools businesses can go for today.
1. Cube
.png?width=287&height=83&name=blue-cube-logo%20(1).png)
Cube is the world’s first spreadsheet-native forecasting tool for businesses of all sizes, combining the most commonly used platforms of Excel and Google Sheets with automations, data syncing, and one-click report generation.
The AI-powered platform cross-reference your company’s financial history, industry best practices, and external events, providing insights on how to plan for the future. Similarly, it also uses AI models to compare how different scenarios will play out, improving the accuracy of your cash forecasting, budgeting, and financial statements.
➡️ Learn more about AI forecasting with Cube ⬅️
2. Datarails

Datarails is anFP&A solution that automates financial reporting and analysis, making it easier to generate accurate forecasts. It uses AI for data consolidation and reporting, and provides finance teams with a centralized platform to manage that data.
While it offers strong capabilities in data management, it may require more customization and setup compared to more user-friendly tools like Cube.
3. Anaplan
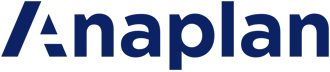
Anaplan supports a wide range of forecasting needs, from finance to supply chain management. Its AI-driven modeling capabilities and flexibility make it suitable for large enterprises with complex forecasting requirements.
However, its complexity can be a drawback for smaller organizations or those without dedicated technical resources.
4. Workday Adaptive Planning
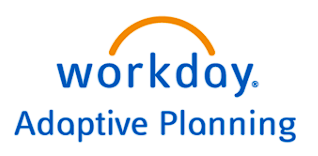
Workday Adaptive Planning straddles both finance and HR, making it a good fit for organizations looking to streamline their processes. It offers solid forecasting and budgeting tools, along with the ability to easily adapt to changing business needs. Its AI capabilities include automating workflows, budgeting, and forecasts, as well as writing up sales and operational plans.
Improve the power of your AI forecasting
Modern forecasting software is designed to give you AI-driven insights, workflow automation, and powerful forecasting capabilities at your fingertips. From one user-friendly dashboard, you can do all your planning and modeling, reporting and analytics, and strategic finance at a higher speed and level of accuracy than traditional methods.
Ready to leap forward in your forecasting capabilities without the steep learning curve? Book a free demo of Cube today.
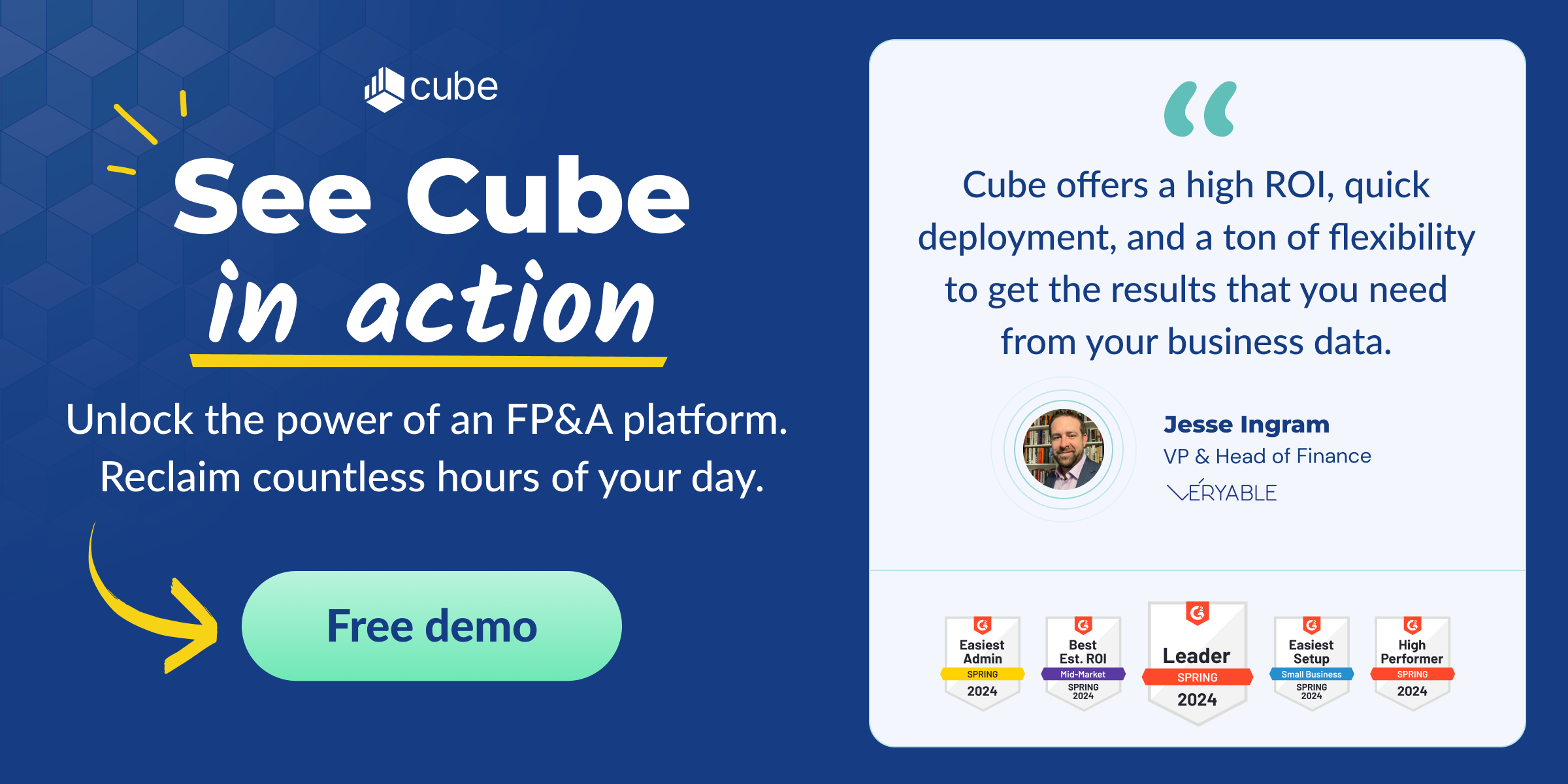