Key takeaways
- Cohorts are customer segments grouped by a shared trait.
- We do cohort analysis to learn more about customer behavior so that we can strategically encourage the behaviors we want to see.
- Acquisition cohorts are grouped by join date/time as customers.
- Behavioral cohorts are clustered by product use behaviors.
- It's best to begin with the end in mind before starting cohort analysis.
What is cohort analysis?
Cohort analysis is when you examine a group of customers who share a specific trait.
Companies do cohort analysis to better understand their revenue.
How does this work?
Because cohort analysis lets companies study a specific group of customers, they can identify patterns in that group's behavior.
An example: knowing why a group churns at a higher rate than average.
Or how they can do better expansion into a specific market.
When we understand our customers, we can better retain and acquire more customers like them.
(This is sometimes called "customer retention analysis," by the way.)
But first, let's define a cohort.
What is a cohort?
A cohort is a group of customers who share a common characteristic.
This could be:
- Location
- Size
- Join time
- Industry
- Plan type
- Time with the company
- Join circumstance
...or any number of factors.
We can account for these in Cube using dimensions.
Why do cohort analysis?
We do cohort analysis to understand how different customer segments behave.
Splitting customers into cohorts helps you better understand their behavior as a group. This means you can also learn how to increase or decrease certain segments of customers.
In other words, it helps you identify trends.
These trends could be (and this list is not exhaustive):
- Churn rates
- Usage patterns
- Expansion rates
Identifying trends at the cohort level is important because they could get washed out at a higher level.
(Or they could be magnified.)
Here's an example:
Company A loses 5% of its monthly revenue to churn. They want to know why, so they do cohort analysis.
They then find that 80% of their churn comes from companies in Cohort B, all SMBs.
So now they know to focus their retention efforts on SMBs.
Telling the story behind the numbers
Cohort analysis lets you understand your revenue from a few different perspectives.
This means that when you tell the story of the company's numbers, you can use cohort analysis to stay honest about the numbers while also giving stakeholders a clearer picture of the reality.
For example, say your company is seeing high churn rates.
You have a hypothesis that the free trial you offered last quarter is the cause of those churn rates, so you do cohort analysis and strip out customers who joined as part of that free trial.
And then you see that your churn rates are on par with your prediction.
Now the numbers look better and you've identified the cause of the problem.
Keep what works and drop what doesn't
Conversely, say you have a specific vertical doing astoundingly well.
You want to replicate that success.
...right?
So you analyze that cohort and look for the factors that led to the current circumstances.
Then, when you're doing scenario planning and budgeting, you can try to reproduce those factors.
You can also do this when looking at cohorts that don't perform well.
And then, you can make the necessary adjustments to your scenarios and budgets.
Acquisition cohorts vs. behavioral cohorts
Two big ways to split customers into cohorts are acquisition and behavioral.
Acquisition cohorts are cohorts based on join time. This kind of cohort helps you identify the who and when.
Here are some examples:
- Joined within 3 months of some big event
- Joined when we offered a free trial
- Joined in Q3 of last year
This kind of cohort is useful when determining at what point your customers tend to churn in the lifecycle.
Acquisition cohort analysis is essential for companies as it reveals the effectiveness of marketing and sales efforts in acquiring new customers over time.
By grouping customers based on their acquisition period, companies can assess the performance of different acquisition channels, optimize marketing spend, and refine targeting strategies to maximize customer acquisition and ROI.
Behavioral cohorts are cohorts based on actions and behavior. This kind of cohort helps you identify the why.
Here are some examples:
- Logs in twice a week
- Doesn't use feature x
- ≤ 200 FTEs
This kind of cohort is helpful when you have a pattern you want to look for or for when you've identified some trait that isn't based on join time.
Behavioral cohort analysis is invaluable for companies as it provides insights into customer behavior patterns, enabling tailored marketing, improved retention efforts, optimized product development, and enhanced customer experiences.
By segmenting customers based on behavior, such as purchase frequency or engagement, companies can personalize approaches, identify dissatisfaction early, prioritize enhancements, and drive satisfaction and loyalty through data-driven decision-making.
6 steps to perform (a simple) cohort analysis
This section will walk you through a simple cohort analysis example.
Like there are plenty of ways to fry an egg, this isn't the only way to perform cohort analysis.
It's not even the most comprehensive way.
But it'll get you started.
Step 1: begin with the end in mind
You could spend all day looking through data if you're anything like us. And while that's valuable for what it could uncover, it might not be the best use of time.
...especially if you're trying to pull together a few KPIs by EOD.
So it's time to begin with the end in mind.
This means: deciding which metrics you're looking to calculate.
Step 2: pull the raw data
Your next step is to pull, gather, cleanse, and organize your raw data.
The data you're looking at might all be in your CRM or ERP. More than likely, though, you'll also want to look at the data that lives wherever you keep your product use metrics.
(We know this step isn't fun. That's why we built Cube.)
It's time for the next step once you've organized that data.
Step 3: identify your dimensions and create cohort identifiers
Identifiers are dimensions in your spreadsheet.
- Location
- Size
- Join time
- Industry
- Plan type
- Time with the company
- Join circumstance
...and so on.
You want to create discrete cohorts as a combination of dimensions.
For this cohort analysis example, Cohort A could be companies that joined in the last 90 days, are based in the Pacific Northwest, and are on your lowest pricing tier.
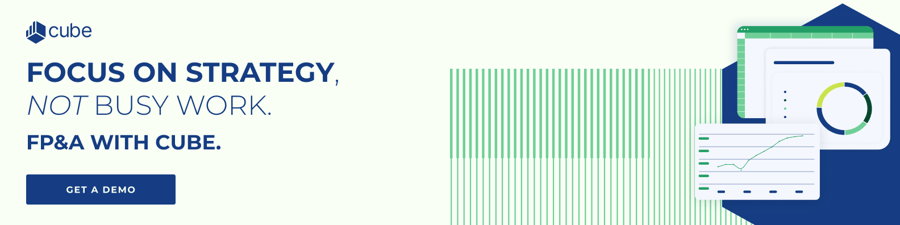
Step 4: slice and dice your cohorts
This is the fun step: pivoting and looking at how the data tell you different stories.
Now that you've identified your cohorts, you can look at the data in several ways.
For the above example, let's say you notice that these companies have a really low gross revenue retention rate.
So you dig into the data a little and notice that half of them started at a higher subscription tier and have been downgraded. Interesting.
You're immediately curious about two things, now:
(1) What happens to those companies in the future? Do they become logo churn, or do they stay with you?
(2) What is it about that group of companies that churned?
To answer either, you create a new cohort, look at the data, and tell a story.
Step 5: gut check
The data tells you a story: does it make sense?
This is a gut-check phase, and it's important to trust your intuition.
For example, if you notice that all the companies who signed up during your free trial period 6 months ago have upgraded to a paid plan...that's suspicious. Free trials are notorious for having higher churn rates, after all.
Step 6: present your findings and suggestions
Now it's time to present the story of the data.
We won't tell you how to present your data to your stakeholders.
However, we did want to give you a strategic tip we've found helpful here at Cube.
It's called inversion. It works like this:
Say your goal is to decrease revenue churn. Instead of focusing on strategies to prevent churn, why don't you identify the factors that lead to revenue churn and then stop doing those things?
It's a simple model and it produces simpler results.
Practical applications of cohort analysis in FP&A
By leveraging cohort analysis effectively, FP&A professionals can uncover hidden opportunities, mitigate risks, and propel their organizations towards sustainable growth. Below are a few practical applications of cohort analysis in FP&A.
Customer Lifetime Value Prediction
Cohort analysis predicts future revenue by segmenting customers based on acquisition or behavior, guiding targeted marketing and retention strategies.
Customer Retention Strategies
Ths behavioral cohort analysis tracks retention rates and churn, enabling tailored initiatives like personalized offers and loyalty programs to enhance customer loyalty.
Product Performance Analysis
Cohort analysis evaluates product adoption, usage patterns, and satisfaction, guiding product development, pricing, and inventory decisions.
Marketing Campaign Effectiveness Evaluation
Cohort analysis assesses campaign performance, comparing response rates and acquisition costs to optimize marketing spend and enhance effectiveness.
Overcoming common pitfalls in cohort analysis
Incorrect Cohort Definition: Defining cohorts incorrectly can skew analysis results and lead to erroneous conclusions. FP&A teams must carefully consider which criteria to use when segmenting cohorts to ensure relevance and accuracy.
This may involve refining cohort definitions based on meaningful segmentation factors such as customer characteristics, acquisition channels, or product usage patterns.
Selection Bias: Selection bias occurs when certain cohorts are disproportionately represented in the analysis, leading to biased insights.
To mitigate selection bias, FP&A professionals should strive for representative sampling when selecting cohorts, ensuring that each cohort is sufficiently large and diverse to draw reliable conclusions.
Ignoring Cohort Dynamics: Cohort dynamics, such as changes in customer behavior over time or external market influences, can significantly impact analysis outcomes. Ignoring these dynamics can lead to misleading conclusions and ineffective strategies.
FP&A professionals should incorporate dynamic factors into cohort analysis by conducting longitudinal studies, tracking cohort performance over extended periods, and considering external variables that may influence cohort behavior.
Overlooking Interpretation Complexity: Cohort analysis results can be complex to interpret, particularly when dealing with multiple cohorts or nuanced trends. FP&A professionals must approach interpretation with caution, taking the time to thoroughly analyze data patterns, identify meaningful insights, and communicate findings effectively to stakeholders.
Visual aids such as charts, graphs, and dashboards can enhance understanding and facilitate clearer communication of cohort analysis results.
Limitations
Cohort analysis is a fantastic tool. But like every tool, it has its limitations.
Cohort analysis won't tell you the underlying cause behind a trend.
For one, cohort analysis helps people find trends in the data. But it's only as good as the imagination.
In Business B's earlier example, knowing that they need to talk with Customer Success isn't helpful if it turns out that Finance and Customer Success don't have a good rapport.
In Business A's example, the analyst might have a blind spot or not think about their onboarding process as influencing churn.
So Business A could spend time perfecting its marketing and sales qualifications and not see a large change in its overall retention.
Cohort analysis takes time.
If you don't start with a specific problem you want to solve, you can spend all day searching through data.
Conclusion: all about cohort analysis
Now you know enough to get started with cohort analysis.
So we're curious...which part of the business are you looking to optimize?
Are you going to dive deep into your 3-month acquisition cohort churn? Or are you curious about performing better with SMBs?
Share this on LinkedIn and tag us to keep the conversation going.
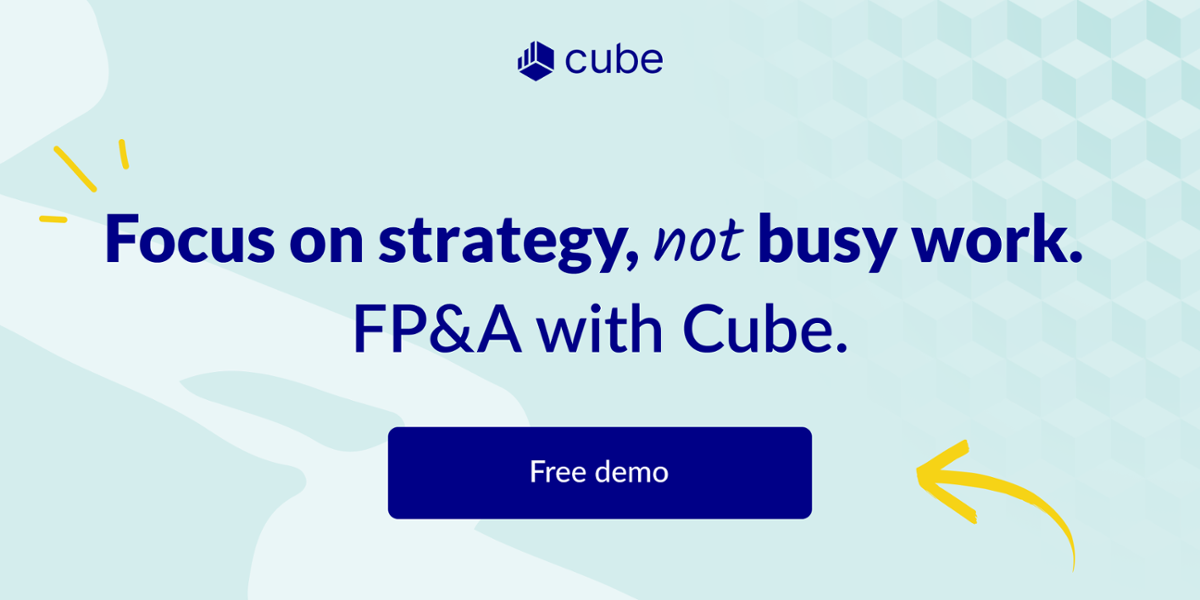