AI data quality: Explaining the feedback loop
Quality data serves as the foundation for effective AI operations, just as accurate financial statements serve as the bedrock for sound business decisions. Clean, well-maintained data allows AI systems to generate reliable insights, while poor-quality data can lead to flawed analysis and misguided recommendations.
But, artificial intelligence isn't just dependent on good data—it can also help maintain it. AI-powered finance and data tools can automatically scan for inconsistencies, flag duplicate entries, and identify missing information. This transforms what was once a manual, error-prone process into a streamlined operation.
In other words, clean data fuels AI performance, and AI, in turn, enhances data quality, creating a feedback loop that drives more reliable decision-making. The impact on financial operations is immediate and measurable. When finance teams work with high-quality data, they can produce accurate forecasts, manage compliance, and spot trends with confidence.
Consider month-end closing: Clean data means faster reconciliation, fewer adjustments, and more time for strategic analysis. Poor data quality, on the other hand, forces teams to spend hours hunting down discrepancies and correcting errors—time better spent on forward-looking analysis and strategic planning.
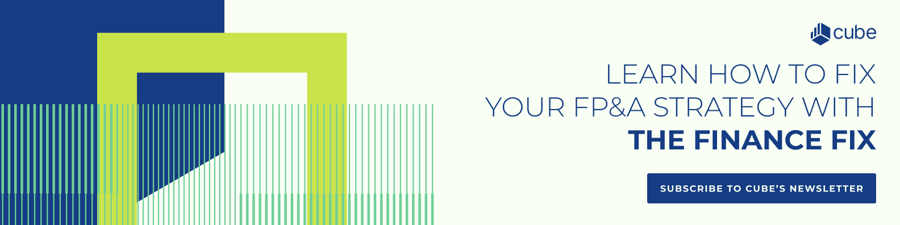
Key components of high-quality data
Let's break down what makes data truly valuable for AI systems.
Accuracy
Clean, error-free data drives reliable AI insights. When your numbers match reality, your AI models can generate dependable forecasts and highlight genuine patterns. Finance teams who maintain accurate data spend less time correcting mistakes and more time acting on meaningful insights. For example, accurate revenue data leads to precise cash flow projections, while errors can trigger a chain reaction of misguided financial decisions.
Consistency
Standardizing data collection and entry processes records all data in the same format and integrates it seamlessly into relational databases. This makes it much simpler to identify trends or anomalies and streamlines the data validation process.
Completeness
Missing data points create blind spots in your AI analysis. Each gap forces your AI system to make assumptions, which can lead to skewed results and unreliable predictions. To make completeness part of your process, establish data collection protocols that capture every relevant data point and routinely audit datasets for gaps. Again, using an AI tool can help by flagging missing entries in real time.
Timeliness
Fresh data powers responsive decision-making—yesterday's numbers won't help you respond to today's challenges. Real-time financial data lets your team adjust forecasts quickly, spot emerging trends, and make faster, more informed decisions. Guaranteeing timeliness is all about automating financial data consolidation so you get synchronized updates rather than relying on manual input. Look for integrations between finance systems and enterprise resource planning platforms that prevent delays and discrepancies.
Relevance
Including unnecessary information doesn't just waste processing power—it obscures important patterns and relationships in your financial data. Keep your data relevant to your specific business objectives. For example, when analyzing departmental performance, include metrics that directly impact bottom-line results and exclude vanity metrics that don't drive decisions.
Best practices for maintaining data quality
While maintaining data quality might seem daunting, following clear practices will keep your AI models running smoothly and your insights reliable. Let's explore the key steps finance teams can take:
1. Conduct proper data collection, labeling, and storage
The foundation of AI success lies in clean, well-organized data. When collecting data, establish clear parameters for what needs to be captured and how. Label each data point consistently—AI models depend on accurate labels to learn and improve. For example, financial transactions should always include standardized details such as currency, date format, and category tags to prevent discrepancies. Additionally, storing data in a structured, centralized system—rather than scattered spreadsheets or disconnected databases—ensures consistency and accessibility.
2. Implement data governance policies
Strong data governance prevents problems before they start. It involves two key aspects:
- Identifying the most relevant data for your business needs
- Structuring it correctly for meaningful analysis
Start by setting specific rules for how your team handles, reviews, and updates data. Governance policies should also define who can access financial data, how to store and share it, and how to make it compliant with legal standards. For instance, role-based access controls can limit sensitive data to only those who need it, while automated audit trails can track any modifications for accountability.
Regular audits and ongoing training will also keep you on top of your governance policies as business needs and regulations evolve. Periodic reviews help catch inconsistencies early, while training ensures that employees stay aligned with best practices and compliance requirements.
3. Set up routine data cleansing
Think of data hygiene like regular maintenance—skip it, and problems compound quickly. Create a clear checklist for each cleaning session, including:
- Remove outdated information
- Fix mismatched numbers
- Delete irrelevant entries
- Verify current data accuracy
- Standardize data formats
- Identify and merge duplicate records
- Fill in missing data points
- Validate data against trusted sources
- Flag anomalies for review
- Track and document changes
Of course, different data types need different cleaning schedules. Sales figures might need daily attention while accounting data works better with monthly reviews. Accurate data is key for reliable forecasting and budgeting, so make sure all of your respective teams are trained to spot and rectify these issues.
4. Effectively consolidate your data
Bring together data from every department—sales, operations, marketing—into one unified system. Financial consolidation tools automatically aggregate data from multiple sources and eliminate discrepancies before humans handle it. They also standardize categorization so you can sort financial data across departments and generate specific or general financial reports without manual adjustments.
Keep your categories practical and purposeful. As departments grow, you'll likely need more specific breakdowns. For example, splitting sales and marketing into separate categories can provide better insights for each team. Regular reviews ensure your categories stay relevant and useful.
5. Prioritize data security
Security protects both your data and your business. Strong security measures help:
- Protect against unauthorized access
- Maintain data integrity
- Build stakeholder confidence
- Support regulatory compliance
Run regular audits to verify system safety and compliance. Use encryption for sensitive information, limit access to authorized team members, and watch for unusual activity patterns. And remember: secure data isn't just protected data—it's data you can trust for making strategic decisions.
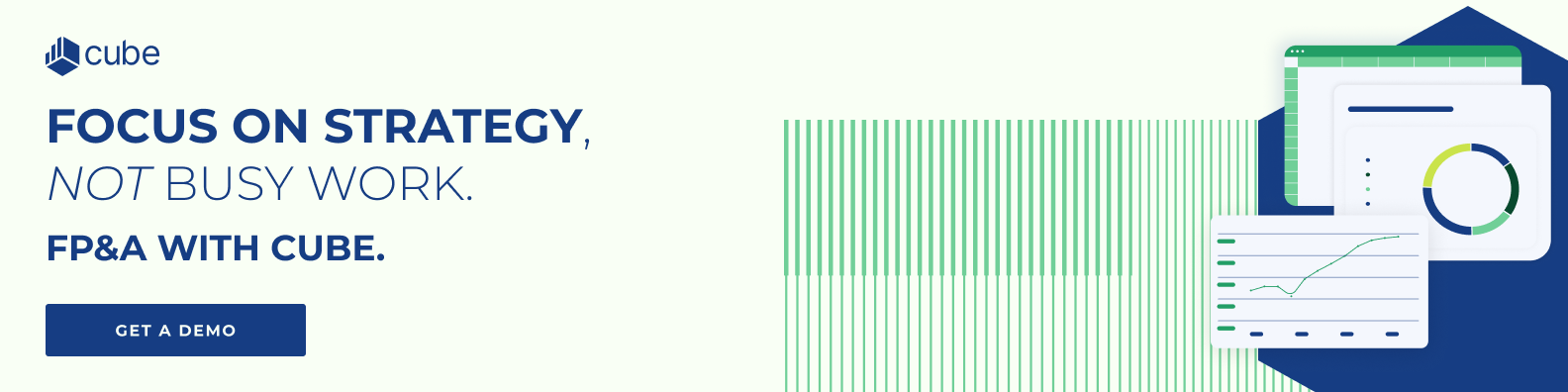
Different ways AI can improve your data quality in finance
Now that you understand how to maintain high-quality data for AI, you can also leverage AI to uphold those standards. AI-powered tools can automate data validation, flag inconsistencies, and improve accuracy—freeing finance teams from time-consuming manual checks. Turn manual processes into automated workflows, convert errors into insights, and transform raw data into reliable decisions. Let's explore how AI strengthens your data quality initiatives.
Data capture and entry
AI makes data capture simple and accurate. Your team can automate collecting information from invoices, contracts, and emails without tedious manual entry. This means fewer errors and more time for strategic work. Just make sure to standardize your data capture methods and formats so data from various sources can be properly integrated.
Error and anomaly detection
Accuracy matters in finance—and AI can prevent costly mistakes by spotting problems faster than any human can. Your finance team gets immediate alerts about duplicate records, outliers, and inconsistencies across massive datasets. For example, when two invoices show matching amounts but different dates, AI can flag the mismatch before it affects your reporting.
With AI monitoring your data in real time, your team stays ahead of irregularities and maintains clean, reliable numbers.
Validation, cleansing, and consolidation
Remember all the validation and data cleansing? AI can help with that. AI tools can automatically validate your data against trusted sources so you know it’s accurate before you start analysis. For example, when expense reports come in, AI cross-checks them against credit card records, instantly flagging discrepancies that could impact budgets or forecasts. Essentially, you’re putting AI in charge of the first pass of data governance, providing accuracy without the burden of manual oversight.
Once the data is in your possession, it’s time to clean it. AI-powered data cleansing can remove outdated entries, standardize formatting, and eliminate inconsistencies—such as merging date formats like "01/01/25" and "Jan 1, 2025" into a single, uniform structure.
AI also strengthens data consolidation by consolidating information across finance, HR, and sales into a single source of truth. With a unified dataset, you get a clearer view of performance metrics, making strategic planning more effective and decisions more confident. This is a key part of AI forecasting, since it predicts the outcome of scenarios based on a maximum amount of data points—something humans can’t match.
Faster analysis and insights
With AI-powered finance tools, your team can get real-time insights instead of waiting for manual analysis. When operational expenses spike, AI alerts you immediately—letting you address issues before they grow. Decision-making improves when you work with current data. Rather than relying on last week's numbers, your team can respond to trends as they emerge. AI processes information continuously, keeping your insights fresh so you can make strategic, informed decisions.
Expedite strategic financial decision-making with advanced AI data quality
Quality data creates better AI models—and better AI models lead to better decisions. Finance teams with clean, accurate data spend less time questioning numbers and more time acting on them. AI can help transform data maintenance from a time-consuming burden into an automated strength, continuously refining datasets to improve accuracy and reduce errors.
Ready to power up your financial data quality with AI? Explore Cube. By automatically identifying inconsistencies and highlighting potential errors, Cube helps you maintain clean, reliable data so you can drive decisions with confidence.
Get ready for a new era of efficient planning with AI. Schedule a demo with Cube today.
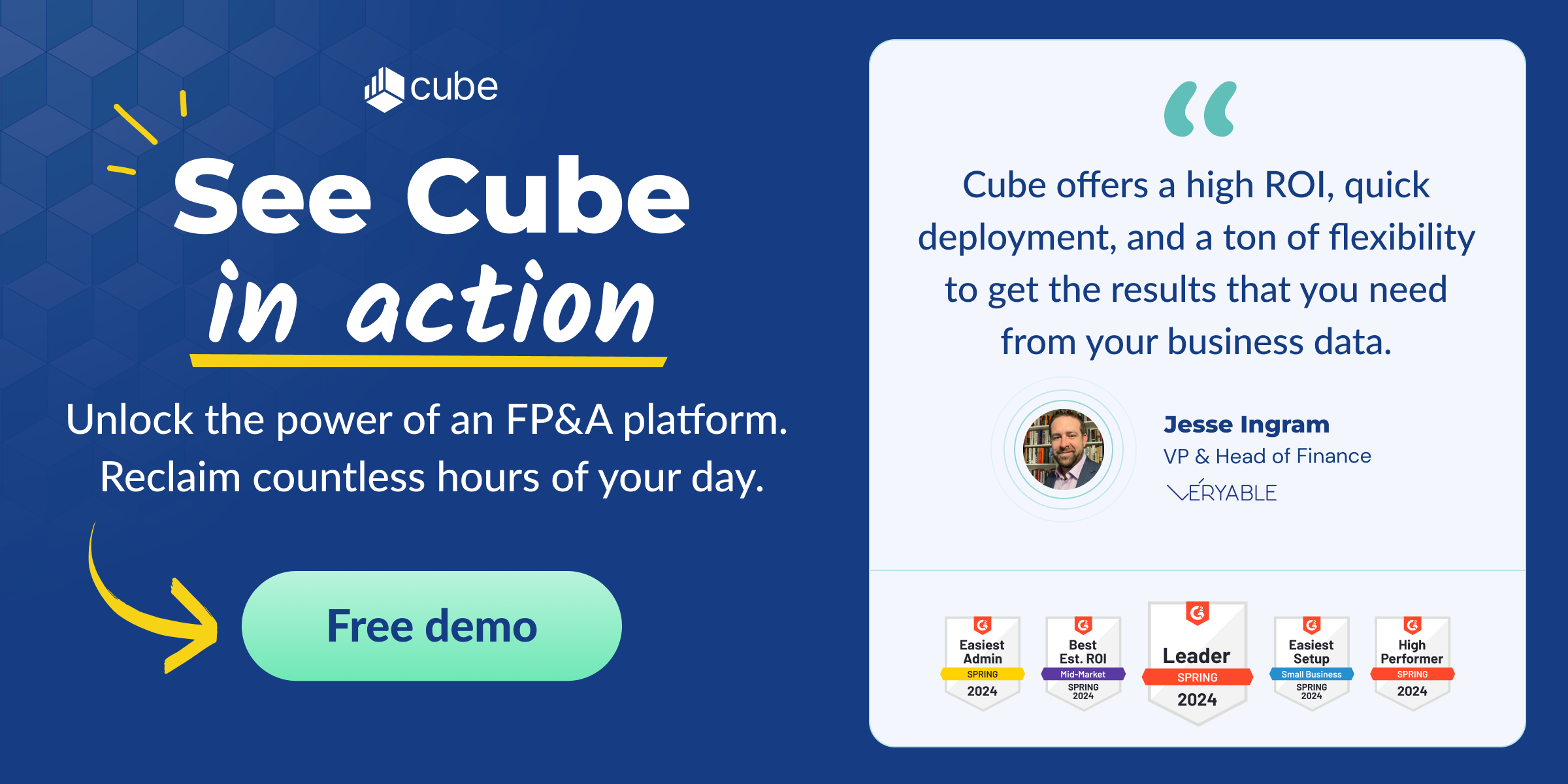