Key takeaways on financial forecasting models
- Financial forecasting models help businesses predict cash flow, expenses, revenues, and workforce needs, enabling informed decision-making.
- Common forecasting models include straight-line, time series, moving averages, and multiple linear regression, each offering unique insights for various business scenarios.
- Financial forecasting improves budgeting accuracy, strengthens strategic planning, and boosts stakeholder trust.
- Using dedicated forecasting software simplifies data handling, ensures accuracy, and enhances collaboration across teams.
What is financial forecasting?
Financial forecasting is where you aim to see into the financial future of your business.
It might sound challenging, but predicting a company's future financial trends and forecasts can be surprisingly accurate. We’re not talking tea leaves here, financial forecasting uses sophisticated analysis of current and historical data to build a picture of what the future might look like.
Financial metrics can feed into financial forecasters, making them useful for business development, financial planning, investment research, and risk management.
It’s a way of working out things like projected investment income, assessing the impact of any internal changes, and anything else that could impact the company’s future performance.
What are financial forecasting models?
Financial forecasting models are tools that businesses use to analyze current and historical data. They help predict financial outcomes for operations and assess the organization’s overall future performance. Common forecasting models include the straight-line method, time series analysis, moving averages, and multiple linear regression. Because they need a hefty amount of data for accurate results, many businesses prefer to use software to simplify the process.
Forecasting models help businesses estimate cash flow, expenses, revenues, workforce needs, and more so they can make informed decisions and achieve their set business goals.
It’s like A Christmas Carol: it involves learning from the past and understanding the present to prepare for the future. But there’s nothing spooky about financial forecasting; with the right tools and data, it’s a powerful way to ensure your business is running a tight ship.
Why are financial forecasting models important?
The benefits of financial forecasting are massive for any FP&A team or CFO, but the wider company may not immediately understand its inherent value and the vital part it plays in business success.
We’ve got that covered. Here are some ways your business can benefit from financial forecasting models.
Improved budgeting
Every business wants and needs a budget that’s rooted in reality. When teams come knocking asking for more resource allocation, financial forecasting can help make those decisions.
With financial forecasting models, the business doesn’t have to guess how much each team needs. They can collect data from past budgets and consider current external factors to determine how much to allocate.
For example, let’s say a manufacturing company has spent $20,000 annually on raw materials for the past three years. Since inflation rates went up by 15%, the team budgeted $23,000 to cover the possible increase in the price of raw materials.
Informed decision-making
If the company is considering a big spend, like an acquisition or partnership, financial forecasting can give the board an idea of what might happen in the months and years following that decision.
They can use the forecasts to build what-if scenarios, identify all possible outcomes and contingencies that may result from the acquisition, and develop strategies to address them.
Financial forecasting becomes a necessary and valuable tool in deciding whether it’s financially feasible to go ahead with a plan or reschedule for another time.
Better stakeholder relations
Investors might want answers if a company changes course on high-stakes decisions like mergers. Financial forecasting is a helpful tool for explaining the wider picture, gaining buy-in, and building trust with investors.
Financial forecasting also helps lenders decide whether a business can safely repay debt. A lender can examine the modeling to determine whether there are any risks associated with giving the business a loan and make an informed decision.
Improves strategic planning
Do you have a strategic plan to put together? Financial forecasting is your new best friend.
Forecasting models can help predict long-term financial health by providing financial insights into current trends, challenges, and growth opportunities. This is perfect for the long-term vision that strategic business planning provides.
Increased efficiency
Forecasting models provide the business’ bigger picture when it comes to financials. They’re a great way of discovering early where the business is underutilizing or overextending resources to stop a nasty surprise from springing up further down the line.
The more efficiently a company can run, the better revenue and profit—so it makes sense to use financial forecasting models to find any holes.
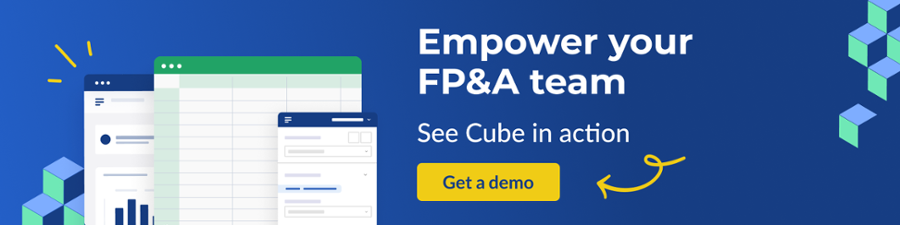
How to do financial forecasting
So forecast models are the bees’ knees, right? But with all the data needed and differing quality and types, that doesn’t make them the most straightforward thing to put together. It’s time for a run-down of how these models work.
There are two different approaches to financial forecasting models: qualitative and quantitative. Let’s take a look at the differences so you can make a decision on which might be best for your company.
Quantitative forecasting models
This is the realm of hard data. Quantitative models are best suited for analyzing a company's performance and anything else that relies on financial statements. With numerical data, statistical analysis, and analysis of older data, these models can pick up on trends and patterns and generate forecasts.
Quantitative forecasting models require a lot of data, and the more data, the better the results will be. Because the data is objective, there are usually fewer errors and biases. As a result, the quality and breadth of the data matter for optimal results.
Qualitative forecasting models
These methods examine data that is difficult to quantify, such as human opinions, market trends, and other subjective factors. They’re also suitable when financial data is lacking, and businesses need to generate estimates.
Qualitative models don’t need as much data to run, making the quality of the data inputted even more crucial. These models are generally less accurate - it’s hard to predict the future as it is - but they’re more flexible, so they work well for new markets and trends.
The best picture will include a mix of the two model types for a complete financial analysis.
Types of financial forecasting methods
No business or industry is the same, so different models exist to help companies with a financial modeling system that suits their needs.
The beauty of these models is that different ones can be used for different scenarios—we’ve provided examples below.
1. Straight-line financial model
Straight-line financial forecasting is a quantitative modeling approach that uses historical financial data and basic mathematical calculations to estimate future growth and predict outcomes.
Most businesses use the straight-line model when they expect an increase in future earnings and want to estimate the percentage increase. It’s particularly useful for making short-term forecasts on revenue, budgets, and sales because it relies solely on the business’s historical performance.
This model typically doesn’t consider external factors like changing market conditions. It assumes static and unchanging market conditions—making it less reliable for building accurate long-term or rolling forecasts.
Straight-line model example
Let’s say you run a SaaS business and want to forecast monthly subscription payment growth. If the total subscription revenue in January 2024 is $50,000 and based on analysis of past data, these payments increased by $1,500 monthly, December’s subscription payments would be:
$50,000 + ($1,500 x 11 months) = $66,500
2. Top-down models
Top-down financial forecasting is a qualitative method that looks at the macro-level view of a market which is then broken down into specific sections like product lines, departments, or regional markets to predict revenue.
Think of it like a sculpture: the original piece of marble is gradually chipped away to form a shape. This is like top-down forecasting, where the business breaks down the bigger picture’s data and forecasts into components where the forecasts are further refined.
Top-down forecasting helps predict sales and revenues in big and complex industries. It’s often complemented by bottom-up forecasting, which is the process in reverse, to further ensure accuracy.
How to calculate this model
To start with top-down forecasting, you need macroeconomic data like Gross Domestic Product (GDP), consumer spending stats, or competitor benchmarking. Initial analysis will get you to the top-level forecasting.
Now, based on the industry analysis, determine your business's market share. If relevant, examine competitors, strategic investments, and SWOT analysis to see how it might evolve.
Now, you can estimate the market sales or revenue forecast by multiplying the estimated market size by the market share. You can then break down that figure into whatever components you need, like product lines or distribution channels.
Creating the forecast allows you to project future sales or revenue for each component by looking at growth rates, market trends, and relevant historical data. Combine those totals for the overall top-down financial forecast.
3. Regression models
Regression forecasting models are statistical tools that use historical data to analyze the relationship between business variables and predict future outcomes. This model establishes a linear relationship between independent and dependent variables to create a trend line.
Businesses use a regression forecasting model because it’s typically easy to implement and offers valuable insights into business trends. If you want some statistical analysis that works out forecasts based on variable changes, then regression models are the way forward.
One popular method is the multiple linear regression model, which looks at the impact of several independent variables on a dependent variable.
It’s a great way to assess the statistical relationship between different components of a business and their impact on revenue. If you have just one independent variable to assess, this is simple linear regression. Simple regression focuses on the relationship between a single independent variable (predictor) and one dependent variable (outcome), such as forecasting sales based on advertising spend alone.
Regression model example
Let’s say a retail company wants to examine the impact of its marketing spend, store size, and average customer income (the independent variables) on its sales (the dependent variable).
A software program can generate the model by gathering historical data on those four variables. If everything’s good and there’s a statistically significant output, you can forecast future sales and make data-driven decisions.
4. Time series models
Any guesses on what these models are based on? That’s right: time. Or more specifically, data points collected over time. Some examples could include GDP, historical sales revenue, or returns on an asset.
They shine by examining which factors influence sales or revenue during a specific period, such as a company's accounting period. Therefore, time series models, such as moving average models, are great for quantifying seasonal patterns in data or identifying any outliers, which can be useful for fraud detection.
It’s like when a tree specialist looks at how many rings a tree has to determine its history and whether weather factors affect its growth up to that point. Time series models look over time to spot trends and patterns to predict future growth.
Time series model example
If a company wanted to predict its monthly sales revenue using a moving average model for the next year, the first step would be to gather all relevant historical data about previous monthly sales revenues from the business.
Using specialist FP&A software, the company can look at the dependencies, trends, and patterns that arise. If everything checks out, it’s possible to estimate future monthly sales from the model.
A typical model for this scenario would be the autoregressive integrated moving average (ARIMA) model. This model is great for short-term forecasting and budgeting, only needs historical data for its output, and cannot consider market curveballs.
5. Scenario-based models
Need a valuation for an upcoming funding round? Then you need a financial model to determine this. One example is the discounted cash flow (DCF) model, which uses the current cash flow to estimate a business's value.
The DCF model works on the basis that the value of a company is the sum of its future cash flow, discounted by the present figure. This makes it a handy tool for CFOs to assess whether a merger or acquisition is a good idea. It's also suitable for investors looking to put their money into a business raising capital and see what return they’ll get.
Scenario-based model example
We’ll use a straightforward merger and acquisition (M&A) example for the discounted cash flow method. If a company wanted to buy a smaller firm, it would look at its statement of cash flows, balance sheet, historical financial performance, growth prospects, and broader industry trends.
The next step is to determine the appropriate discount rate or rate of return for an investor. But how to determine the discount rate? A weighted average cost of capital (WACC) usually does the trick as it factors in debt and equity financing.
With the discount rate, the company can return the projected future cash flows to the present. If the final figure is lower than expected, that might give a company pause to consider whether that firm is a good buy.
6. Three-statement financial model
This dynamic financial model links the holy trinity of the cash flow statement, balance sheet, and income statement. By examining how the three financial statements interact, finance pros can assess various factors such as the business’s profitability, solvency, and cash generation.
The three-statement model is great for setting budgets and internal planning to allocate resources more effectively. It’s also good for assessing how well parts of the business are performing financially and whether any tweaks will improve profitability.
Companies can also use three-model statements to determine whether a planned expansion, like an investment or acquisition, is financially viable.
How to calculate the three-statement model
The process has a few steps with the pro forma financial statements, but the payoff is worth it.
- Starting off with the income statement projections, forecast the future revenue, cost of goods sold, and operating expenses based on historical data. This will produce the estimates needed for future profits and income.
- Now it’s time for the balance sheet forecast. Looking at the projected income statement to work out forecasts on future assets, liabilities, and equity, will give a better picture of the company’s projected financial position.
- The third and final piece of the puzzle is the cash flow statement. Using the projected income statement and balance sheet, you can forecast the future cash flows from any operations and investing activities.
With three separate forecasts required for this model, software programs can make the whole process much smoother and quicker for finance teams.
Have you got everything together? Then, you have a comprehensive overview of any planned expansion's impact on the business’s finances. This can help a company decide whether sufficient money is coming in, whether it needs financing, or whether optimizing the capital structure is better before pressing ahead.
These models serve different purposes and yield different results. The key to success is good-quality data, which can make or break the outputs.
7. Bottom-up financial model
The bottom-up financial forecasting model projects micro-level inputs to predict an organization’s financial performance for a set of years or a single year. Businesses use it to estimate future outcomes using lower-level variables such as individual sales rep performance.
With bottom-up forecasting, organizations need to break down the business into components that drive its growth, revenue, and profits, collect historical data on each component, and then build a consolidated forecast.
Bottom-up forecasting is quite objective. It considers the actual capability of individual teams and departments based on past performance, which enables the organization to build more accurate projections.
Bottom-up model example
A growing SaaS business may want to forecast revenue for the next year and have five sales reps who sell software subscriptions. The company breaks down key revenue drivers: the number of sales reps, deal size, quota per sales rep, and conversion rates.
The company will collect historical data on each sales rep's performance. Let’s say Rep 1 sold $50,000 from two deals, and Rep 2 sold $90,000 from four deals. Then, the company will get data on other factors affecting their performance, like marketing costs and economic conditions.
Using this information, the company can estimate that Rep 1 will sell $70,000 in the next year and Rep 2 will sell $120,000, given that marketing spending increases and economic conditions are good.
8. Correlation forecasting model
The correlation forecasting model examines the strength of the relationship between two variables (dependent and independent) to predict future outcomes. It enables businesses to identify key drivers between variables, such as sales and consumer income.
A correlation model monitors variations between two variables and quantifies their similarities. FP&A teams plot the data points using a scatter diagram, with the dependent variable on the y-axis and the independent variable on the x-axis.
A line of best fit indicates the strength of the correlation between variables and the correlation coefficient, with the correlation being stronger when it’s closer to 1 or -1. 1 represents a perfect positive correlation between variables, and -1 indicates a perfect negative relationship.
Correlation forecasting example
If you own an ice cream company and want to forecast summer demand to adjust production and supply, start by identifying two key variables: ice cream sales (the dependent variable) and temperature (the independent variable). By analyzing the relationship between these, you can better predict demand and manage inventory and resources.
You’ll gather historical data on daily ice cream sales and corresponding temperature readings over a time period. Then, plot the data on a scatter graph and calculate the correlation. If the result is closer to +1, it means there’s a strong positive correlation between sales and weather.
9. Delphi forecasting model
Delphi forecasting models take a qualitative approach. They involve using a panel of verified experts to generate insights, opinions, and consensus on a specific topic through a series of feedback rounds and questionnaires.
It was first used during the Cold War to forecast the effect of technology on warfare. This method hinges on the school of thought that group knowledge is more accurate than individual opinions.
The Delphi model follows a four-step process. It starts with the experts answering open-ended questions to provide their suggestions, predictions, or insights. A facilitator compiles all responses and shares them with the experts while keeping them anonymous.
At the review stage, the experts will review the summary of answers and revise their suggestions or predictions based on the group response. This continues until the group reaches a general consensus.
Delphi forecasting example
Hospitals commonly use Delphi forecasting to predict clinical developments, develop consensus around clinical issues, and establish treatment protocols and best practice guidelines.
A real-life example is hospitals using the model during the COVID-19 pandemic to forecast ICU bed availability, medical staff capability, ventilators, and the number of patients they might treat by gathering expert opinions from a panel of professionals. The general consensus was then used to make informed decisions on how to handle emergency patients, extra equipment to get, etc.
10. Statistical forecasting model
Statistical forecasting models (or quantitative forecasting models) analyze business statistics to determine the relationship between variables and predict future outcomes based on their correlation. The models work using key components like trends, time series data, and seasonality to compare how a business operates similarly to other businesses in its sector.
Statistical models work best when external factors have a limited impact on the quality of data, when past data is relatively stable, and when the business expects future outcomes to follow the same patterns. They are also best for the organization’s short—to medium-term forecasting.
There are various statistical forecasting models. Popular models include regression analysis, moving averages, straight-line, and exponential smoothing.
Statistical forecasting example
Let’s say you manage a small bookshop and want to build a weekly sales forecast using a three-week moving average. Past sales are for weeks one (100 books), two (120 books), and three (110 books).
To calculate sales in week four, calculate the average of the first three weeks: 100 + 120 + 110/3 = 110. Based on past sales trends, you can expect to sell 110 novels in week four.
Impact of external events on financial forecasting models
Financial forecasting models are essential tools in today's changing business environment for firms trying to manage uncertainty and make long-term plans. These models, however, are not immune to the effects of outside factors that have the potential to upset markets and economies.
Financial forecasts have major hurdles during economic downturns and recessions, which are characterized by decreased GDP growth and rising unemployment rates.
Forecasting is made more difficult by market volatility and uncertainty since shifting stock prices and investor mood can have unanticipated results. Financial forecasting models get significantly more complex when geopolitical events, such as trade disputes and geopolitical tensions, occur because they have the potential to alter global supply chains and currency exchange rates.
By adopting flexible and adaptive forecasting approaches, incorporating scenario planning, and risk mitigation strategies, businesses can enhance the resilience of their forecasting models and make more informed decisions in an ever-changing world.
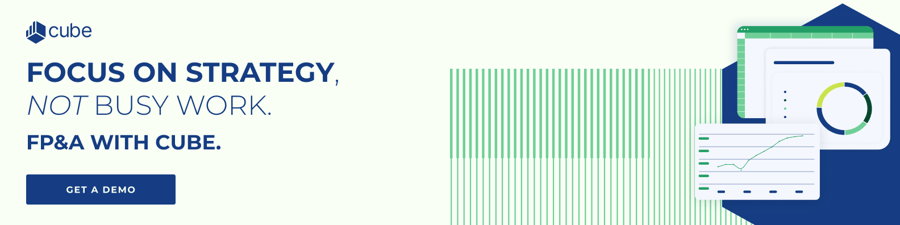
Industry-specific considerations in financial forecasting models
Each industry presents its unique set of challenges and dynamics, shaping the intricacies of financial forecasting models. In this section, we'll explore industry-specific considerations in financial forecasting models, focusing on a few major sectors.
Healthcare sector
In the healthcare sector, financial forecasting models must navigate complex factors such as regulatory changes, reimbursement structures, and evolving patient demographics.
Key considerations include:
- Regulatory compliance: Healthcare organizations must account for frequently changing regulations, such as billing requirements and healthcare policies, which can impact cost structures and reimbursement rates. Financial forecasts should factor in these regulatory shifts to maintain compliance and predict how they might affect revenue cycles.
- Demand forecasting: Patient demand can fluctuate based on demographic changes, seasonality (e.g., flu season), and unexpected events like pandemics. Forecasting demand accurately helps allocate resources efficiently, ensuring that facilities are neither under nor over-supplied.
- Revenue cycle management: Revenue cycles in healthcare are complex, involving multiple payment sources (private insurance, government programs, patient out-of-pocket). Forecasting models must account for potential reimbursement delays and fluctuating payment rates to manage cash flow effectively.
Hospitality and tourism
The hospitality and tourism industry is highly sensitive to economic cycles, consumer trends, and geopolitical events. Financial forecasting models in this sector should factor in:
- Seasonality: Travel patterns often follow seasonal trends. Forecasts must incorporate these predictable fluctuations to ensure staffing, supply levels, and marketing efforts align with demand changes.
- Economic indicators: Factors like consumer spending power, unemployment rates, and inflation can influence tourism. Tracking these indicators helps businesses adjust pricing strategies and anticipate shifts in booking volumes.
- Market segmentation: Different customer segments (e.g., business travelers and leisure tourists) respond differently to economic and market conditions. Accurate forecasting requires an understanding of these segments’ specific needs and preferences to create tailored promotions and pricing strategies.
SaaS industry
In the Software as a Service (SaaS) industry, financial forecasting models encounter unique challenges shaped by subscription-based revenue models, customer acquisition costs, and churn rates.
Key considerations include:
- Customer acquisition costs: SaaS companies often invest heavily in acquiring customers, so forecasting should factor in the cost per acquisition versus the long-term value of each customer. This helps determine marketing budgets and assess whether growth strategies are sustainable.
- Monthly recurring revenue (MRR): MRR is a key metric in the SaaS model, and forecasts should include new subscriptions, upgrades, downgrades, and cancellations to accurately predict monthly revenue flows.
- Churn rate: Customer retention and churn directly impact revenue stability. High churn rates signal frequent customer loss, which reduces recurring revenue and affects growth projections. By analyzing churn trends, forecasting models can help identify at-risk customer segments and guide retention strategies, ensuring more consistent revenue streams and improving the accuracy of long-term projections.
- Expansion revenue: Upselling and cross-selling existing customers add a valuable revenue stream. Forecasting should anticipate the likelihood of upgrades or add-on purchases, which can boost revenue without additional acquisition costs.
Manufacturing industry
Financial forecasting models in the manufacturing industry grapple with operational complexities, supply chain dynamics, and inventory management challenges. Forecasting models should include:
- Production costs: Raw material prices, labor costs, and energy expenses significantly impact production costs. Forecasting these elements helps manage profitability and anticipate adjustments to production pricing or output.
- Inventory management: Efficient inventory forecasting ensures that production runs smoothly without overstocking, which ties up capital, or understocking, which disrupts the supply chain. Models need to account for lead times and demand variability.
- Production capacity: External factors like supplier reliability, geopolitical events, and natural disasters can disrupt supply chains. Models should incorporate risk factors to plan for potential disruptions and build resilience through alternative sourcing or inventory buffers.
- Supply chain risks: External factors like supplier reliability, geopolitical events, and natural disasters can disrupt supply chains. Models should incorporate risk factors to plan for potential disruptions and build resilience through alternative sourcing or inventory buffers.
Benefits of financial modeling software
An excellent software program for financial modeling will enhance your team’s efficiency, accuracy, and decision-making progress. Want to free them up to focus on the big things? Then software programs are the way to go.
Here are some more benefits of using software for complex financial modeling:
Compare multiple models
Get ready for some advanced analytics. Using software programs makes comparing qualitative and quantitative models a breeze, saving your team time and resources.
Evaluating performance and analyzing different financial scenarios has never been easier. Comparing several financial models at once can help spot risks before they happen, notice new opportunities, and improve decision-making.
Customizable
Every organization is different, so you’ll need tailored solutions to showcase the financial models. The software makes custom calculations, templates, and reports simple to tailor to any business size or industry.
This custom approach can be helpful when a company is scaling, as the models can grow with the company instead of staying static and needing reworking.
Automation and speed
Without FP&A software, an FP&A team or specialist would need to assemble an Excel spreadsheet from scratch (as every company’s financial system is unique).
After that, the data must be manually aggregated and inputted into the modeling spreadsheet - a time-consuming practice that can lead to mistakes.
The software eliminates the admin around financial modeling and automates the whole process. Many software programs use machine learning and AI to pull and analyze the data for financial modeling, saving your team hours of time and reducing busy work.
Seamless data integration
Say goodbye to endless Excel spreadsheets that are constantly outdated. With software, you can easily import, handle, and analyze large amounts of data from various sources.
The software then processes the data in real-time, so you’re never left making analyses from incorrect or old data sources. It’s a win-win.
Collaborative opportunities
Have you ever had a situation where someone made a change that broke the whole model? With modern software, that’s a thing of the past.
Cloud-based software lets FP&A teams collaborate on one or multiple financial forecasting models with regular updates and version control. This way, everyone’s working with the most up-to-date data and assumptions.
Best financial forecasting and modeling software
If you want to optimize your financial forecasting process and build more accurate forecasts, these are the best software to use.
1. Cube

Cube is a cloud-based FP&A platform that helps companies hit their numbers without having to sacrifice their spreadsheets. Cube’s AI-powered platform works right in Excel and Google Sheets to eliminate the inefficiencies and complexities often associated with traditional FP&A tools.
Many high-growth companies like Instride and Figment use Cube for their FP&A needs, including projects that require coordination across various teams like reporting, forecasting, and budgeting.

Even more importantly, Cube was founded by a former CFO, building it with the problems and use cases of FP&A in mind from the start. The platform enables you to easily:
- Access and edit on demand: Digest data, present insights, and pivot with the agility you need in your spreadsheets, in dashboards, and on the web.
- Automated data consolidation: Connect real-time data from numerous sources for automated rollups and drilldowns.
- Smart forecasting: Jumpstart planning tasks by creating an AI-proposed forecast that you can use as a starting point or benchmark for your existing plans.
- Proactive variance analysis: Automatically highlight financial variances and give your team the info they need faster. AI offers you the first analysis of your variances so you can decide where to dig in deeper to help get the company back on track.
- Endless integrations: Seamlessly integrate tools like spreadsheets (Google and Excel), accounting & finance, HR, ATS, billing & operations, sales & marketing, and business intelligence.
- Customizable dashboards: Build and share customizable dashboards.
- Native Excel and Google Sheets integration: Leverage compatible and bi-directional native integration with any spreadsheet.
- Multi-currency support: Evaluate your financials in both your local and reporting currencies.
- Drill down and audit trails: With just one click, you can access the transactions and history behind a single data cell.
Cube gives finance teams complete control over their forecasting process while allowing them to work better in tools they’re already familiar with—Excel and Google Sheets.
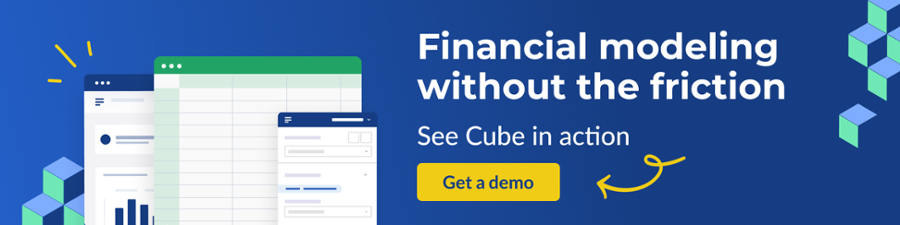
2. Workday Adaptive Planning

Workday Adaptive Planning is enterprise performance management (EPM) software that enables organizations to leverage AI and ML capabilities in their budgeting, planning, and modeling. It lets businesses build financial, workforce, and operational plans and streamline their close and consolidation process.
Its forecasting software helps businesses build daily, monthly, quarterly, or rolling forecasts. It provides one source of data and integrated workflows, allowing different departments or teams to build forecasts collaboratively.
The platform allows users to create and compare multiple what-if scenarios based on forecasts, integrate other backend enterprise software, import their ERP data, and build up-to-date models.
Read our complete Workday review.
3. Anaplan

Anaplan offers a connected planning tool for finance, sales, HR, and supply chain teams. Its financial planning and analysis platform enables businesses to forecast and build multi-dimensional models to project potential outcomes based on real-time metrics.
The software lets businesses anticipate complex market changes by analyzing multiple variables such as product, location, time, expense, customer, and currency.
Anaplan also offers an intelligent predictive planning platform that allows users to generate insights and intent data from different sources and integrates with Salesforce, Microsoft, Tableau, and other business platforms.
Read our complete Anaplan review.
4. Planful

Planful has an FP&A platform that offers pre-built templates for financial forecasting and allows businesses to leverage AI and ML functionalities to automatically collect, store, and categorize data, identify abnormalities or trends, and build accurate forecasts.
It provides a single central platform on which businesses can store their financial data and teams can collaborate on forecasts, track actuals, and update financial plans, budgets, and forecasts. FP&A teams can build baseline forecasts across multiple business units with other departments.
Planful’s rolling forecasts tool lets businesses account for shifting trends and ongoing market volatility by creating forecasts with adjustable key drivers. The software integrates with Salesforce, Oracle, Workday and more.
Read our complete planful review.
The bottom line on financial forecasting models
Financial forecasting models are essential tools for businesses to predict future performance, make informed decisions, and remain competitive. By using these models, companies can better allocate resources, anticipate risks, and align their strategies with market trends.
Choosing the right software is important to streamline the forecasting process. With a user-friendly platform, your team can focus on the insights that matter most and make proactive, data-driven decisions.
Cube sets itself apart from other financial planning tools by keeping you in your familiar environment—Excel and Google Sheets—while enhancing your forecasting capabilities for better accuracy and collaboration.
Ready to boost your forecasting? Book a free demo today.
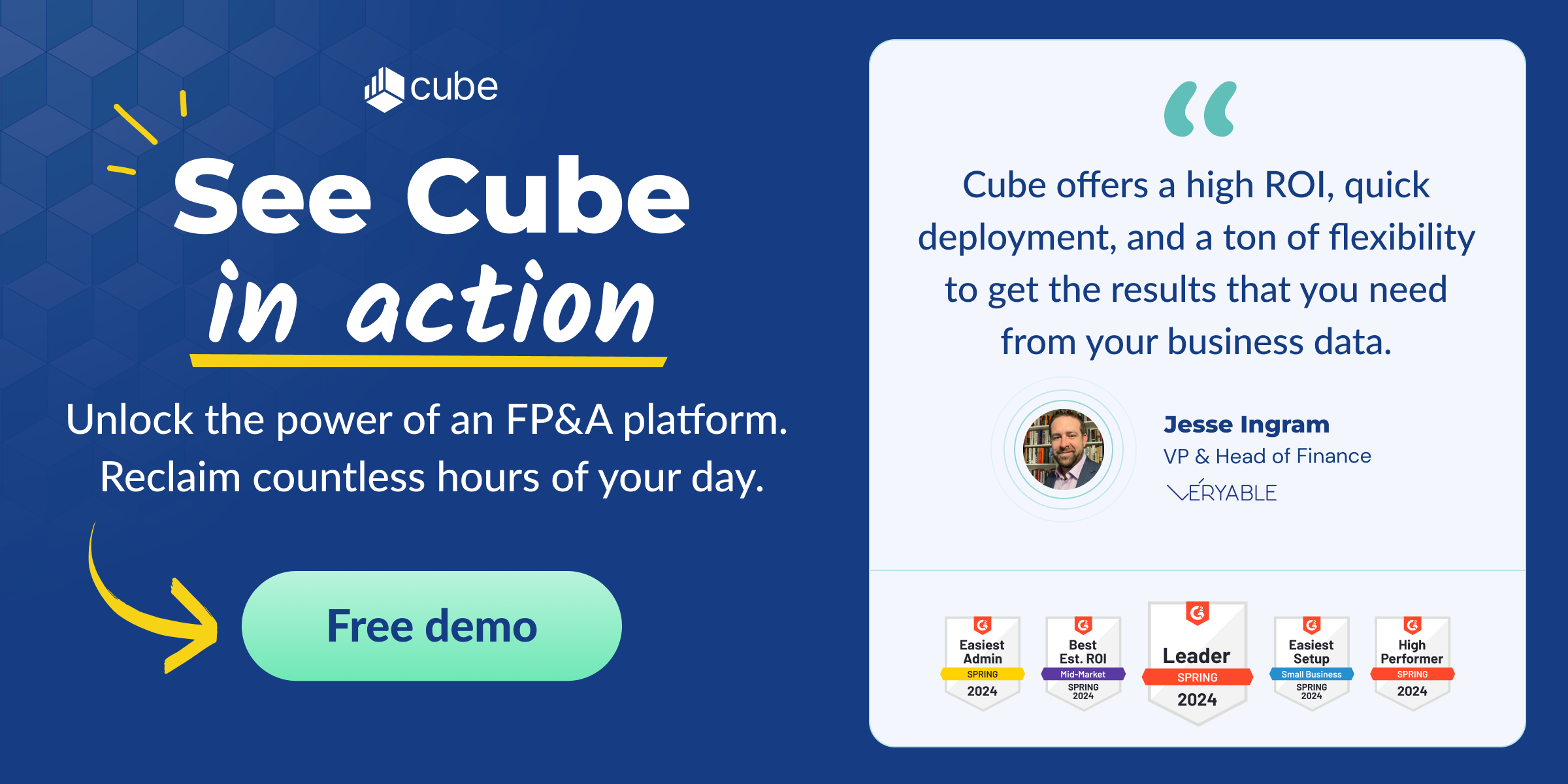